Periodic Scientific Seminar, Department of Computer Science (Faculty of IT), University of Technology
The December periodic seminar was held on December 23, under the coordination of Assoc. Prof. Nguyen Thi Nhat Thanh. The session focused on Artificial Intelligence and Remote Sensing, continuing the theme from the previous month. It featured two distinguished speakers: Assoc. Prof. Chanh Kieu from Indiana University Bloomington, USA, and Asst. Prof. Harry Nguyen from University College Cork – National University of Ireland. The event attracted researchers, students, and other attendees interested in these cutting-edge topics.
In the first segment, Assoc. Prof. Chanh Kieu discussed the challenges and strategies for retrieving tropical cyclone (TC) intensity using gridded climate reanalysis data. He employed two widely used deep learning architectures—Convolutional Neural Networks (CNNs) and Vision Transformers (ViTs)—to demonstrate their capabilities in estimating TC intensity with a mean absolute error of ±7.5 knots. His analysis highlighted the sensitivity of TC intensity retrieval to data sampling methods, especially for convolutional architectures. By contrast, transformer-based approaches displayed greater robustness to variations in data sampling, positioning them as a promising solution for future TC intensity retrieval and TC climate downscaling applications.
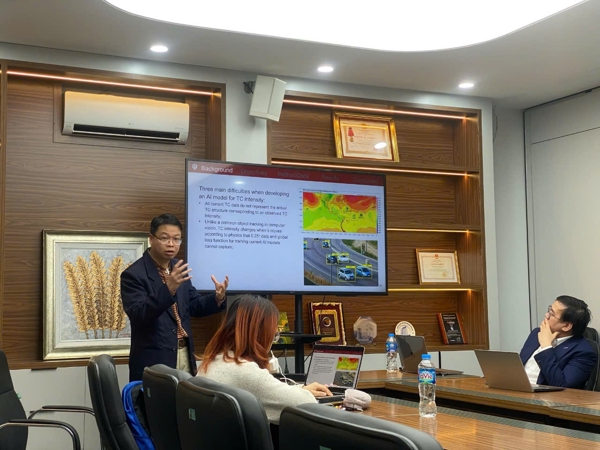
Assoc. Prof. Chanh Kieu presenting on "Retriveving tropical cyclone intensity from climate output: AI approaches and challenges."
In the second segment, Asst. Prof. Harry Nguyen introduced his work on Reliable AI for Carbon Stock Estimation on a Global Scale Using Aerial and Satellite Imagery. Addressing the urgent need to mitigate climate change, his research focuses on developing reliable AI systems to assess global carbon stocks, supporting climate action initiatives. This involves key AI tasks such as detecting tree coverage across diverse forest ecosystems and estimating carbon stock from large volumes of high-resolution imagery. These AI-driven methods offer a superior alternative to traditional approaches, which are labor-intensive, time-consuming, costly, and often invasive. The research integrates various remote sensing data sources—from aerial to satellite imagery—into a standardized and harmonized dataset comprising 107,856 high-resolution images. This standardization ensures consistent data quality and properties across platforms. A novel technique for tree semantic segmentation was introduced, providing a more effective method for large-scale tree coverage estimation compared to conventional tree crown delineation. The study also showcased an interactive AI system designed for exploring high-vegetation patches and estimating carbon stocks on a global scale.
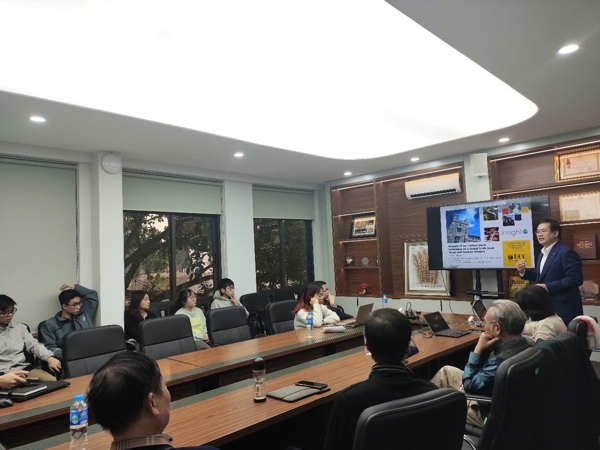
Asst. Prof. Harry Nguyen presenting on "Reliable AI for Carbon stock estimation on a global scale from aerial and satellite imagery."
The presentations were highly successful, offering valuable insights into the application of AI in addressing global challenges such as climate change and natural disaster management.